Configuration
The YAML configuration format runs each prompt through a series of example inputs (aka "test case") and checks if they meet requirements (aka "assertions").
Assertions are optional. Many people get value out of reviewing outputs manually, and the web UI helps facilitate this.
Example
Let's imagine we're building an app that does language translation. This config runs each prompt through GPT-3.5 and Gemini, substituting language
and input
variables:
prompts: [prompt1.txt, prompt2.txt]
providers: [openai:gpt-4o-mini, vertex:gemini-pro]
tests:
- vars:
language: French
input: Hello world
- vars:
language: German
input: How's it going?
For more information on setting up a prompt file, see input and output files.
Running promptfoo eval
over this config will result in a matrix view that you can use to evaluate GPT vs Gemini.
Use assertions to validate output
Next, let's add an assertion. This automatically rejects any outputs that don't contain JSON:
prompts: [prompt1.txt, prompt2.txt]
providers: [openai:gpt-4o-mini, vertex:gemini-pro]
tests:
- vars:
language: French
input: Hello world
assert:
- type: contains-json
- vars:
language: German
input: How's it going?
We can create additional tests. Let's add a couple other types of assertions. Use an array of assertions for a single test case to ensure all conditions are met.
In this example, the javascript
assertion runs Javascript against the LLM output. The similar
assertion checks for semantic similarity using embeddings:
prompts: [prompt1.txt, prompt2.txt]
providers: [openai:gpt-4o-mini, vertex:gemini-pro]
tests:
- vars:
language: French
input: Hello world
assert:
- type: contains-json
- type: javascript
value: output.toLowerCase().includes('bonjour')
- vars:
language: German
input: How's it going?
assert:
- type: similar
value: was geht
threshold: 0.6 # cosine similarity
To learn more about assertions, see docs on configuring expected outputs.
Import providers from separate files
The providers
config property can point to a list of files. For example:
providers:
- file://path/to/provider1.yaml
- file://path/to/provider2.json
Where the provider file looks like this:
id: openai:gpt-4o-mini
label: Foo bar
config:
temperature: 0.9
Import tests from separate files
The tests
config property takes a list of paths to files or directories. For example:
prompts: prompts.txt
providers: openai:gpt-4o-mini
# Load & runs all test cases matching these filepaths
tests:
# You can supply an exact filepath
- tests/tests2.yaml
# Or a glob (wildcard)
- tests/*
# Mix and match with actual test cases
- vars:
var1: foo
var2: bar
A single string is also valid:
tests: tests/*
Or a list of paths:
tests: ['tests/accuracy', 'tests/creativity', 'tests/hallucination']
We also support CSV datasets from local file and Google Sheets.
Import vars from separate files
The vars
property can point to a file or directory. For example:
tests:
- vars: path/to/vars*.yaml
You can also load individual variables from file by using the file://
prefix. For example:
tests:
- vars:
var1: some value...
var2: another value...
var3: file://path/to/var3.txt
Javascript and Python variable files are supported. For example:
tests:
- vars:
context: file://fetch_from_vector_database.py
This is useful when testing vector databases like Pinecone, Chroma, Milvus, etc.
Javascript variables
To dynamically load a variable from a JavaScript file, use the file://
prefix in your YAML configuration, pointing to a JavaScript file that exports a function.
tests:
- vars:
context: file://path/to/dynamicVarGenerator.js
dynamicVarGenerator.js
receives varName
, prompt
, and otherVars
as arguments, which you can use to query a database or anything else based on test context:
module.exports = function (varName, prompt, otherVars) {
// Example logic to return a value based on the varName
if (varName === 'context') {
return {
output: `Processed ${otherVars.input} for prompt: ${prompt}`,
};
}
return {
output: 'default value',
};
// Handle potential errors
// return { error: 'Error message' }
};
This JavaScript file processes input variables and returns a dynamic value based on the provided context.
Python variables
For Python, the approach is similar. Define a Python script that includes a get_var
function to generate your variable's value. The function should accept var_name
, prompt
, and other_vars
.
tests:
- vars:
context: file://fetch_dynamic_context.py
fetch_dynamic_context.py:
def get_var(var_name, prompt, other_vars):
# Example logic to dynamically generate variable content
if var_name == 'context':
return {
'output': f"Context for {other_vars['input']} in prompt: {prompt}"
}
return {'output': 'default context'}
# Handle potential errors
# return { 'error': 'Error message' }
Avoiding repetition
Default test cases
Use defaultTest
to set properties for all tests.
In this example, we use a llm-rubric
assertion to ensure that the LLM does not refer to itself as an AI. This check applies to all test cases:
prompts: [prompt1.txt, prompt2.txt]
providers: [openai:gpt-4o-mini, vertex:gemini-pro]
defaultTest:
assert:
- type: llm-rubric
value: does not describe self as an AI, model, or chatbot
tests:
- vars:
language: French
input: Hello world
assert:
- type: contains-json
- type: javascript
value: output.toLowerCase().includes('bonjour')
- vars:
language: German
input: How's it going?
assert:
- type: similar
value: was geht
threshold: 0.6
You can also use defaultTest
to override the model used for each test. This can be useful for model-graded evals:
defaultTest:
options:
provider: openai:gpt-4o-mini-0613
YAML references
promptfoo configurations support JSON schema references, which define reusable blocks.
Use the $ref
key to re-use assertions without having to fully define them more than once. Here's an example:
prompts: [prompt1.txt, prompt2.txt]
providers: [openai:gpt-4o-mini, vertex:gemini-pro]
tests:
- vars:
language: French
input: Hello world
assert:
- $ref: '#/assertionTemplates/startsUpperCase'
- vars:
language: German
input: How's it going?
assert:
- $ref: '#/assertionTemplates/noAIreference'
- $ref: '#/assertionTemplates/startsUpperCase'
assertionTemplates:
noAIreference:
type: llm-rubric
value: does not describe self as an AI, model, or chatbot
startsUpperCase:
type: javascript
value: output[0] === output[0].toUpperCase()
tools
and functions
values in providers config are not dereferenced. This is because they are standalone JSON schemas that may contain their own internal references.
Multiple variables in a single test case
The vars
map in the test also supports array values. If values are an array, the test case will run each combination of values.
For example:
prompts: prompts.txt
providers: [openai:gpt-4o-mini, openai:gpt-4]
tests:
- vars:
language: [French, German, Spanish]
input: ['Hello world', 'Good morning', 'How are you?']
assert:
- type: similar
value: 'Hello world'
threshold: 0.8
Evaluates each language
x input
combination:
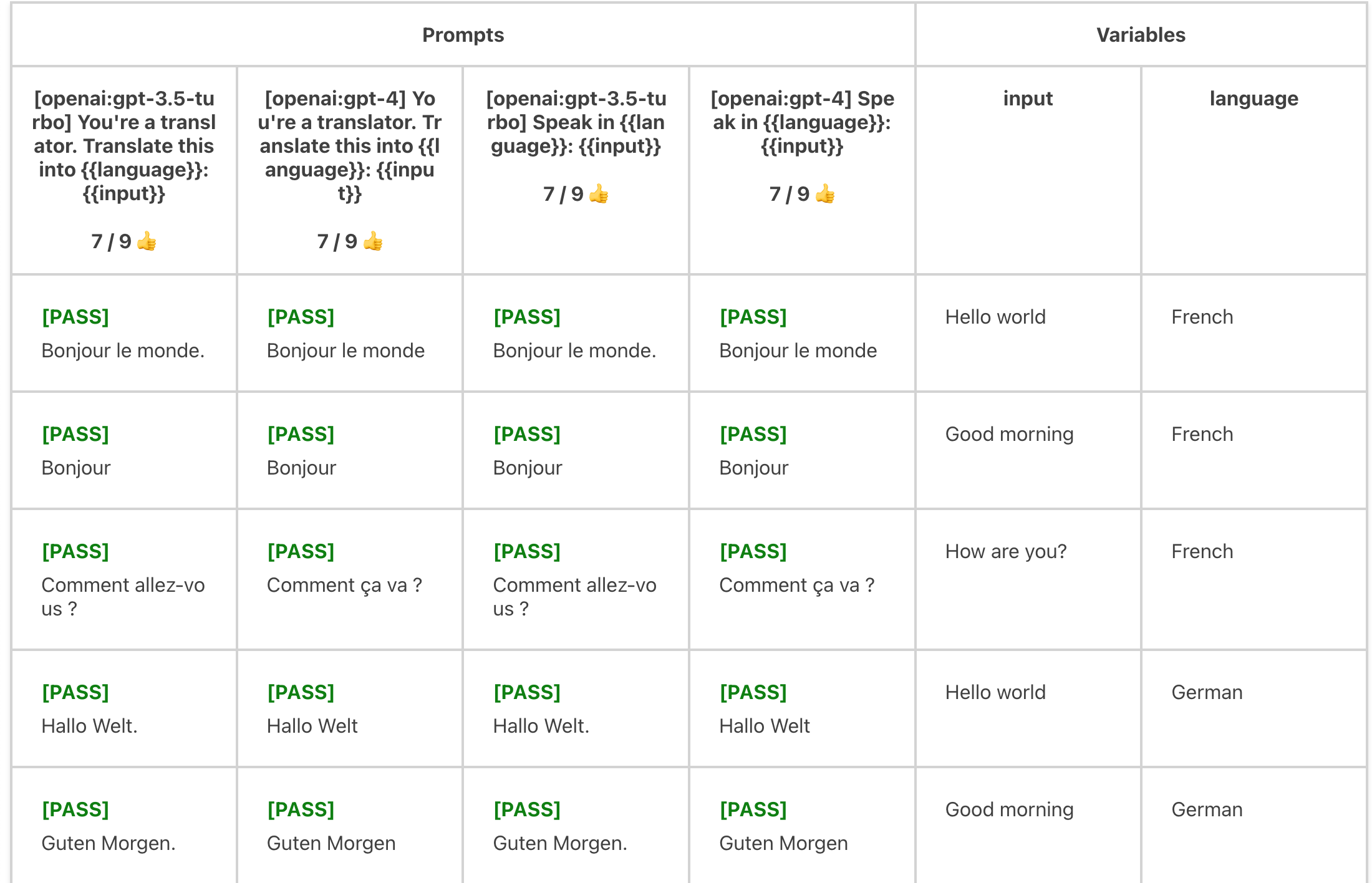
Vars can also be imported from globbed filepaths. They are automatically expanded into an array. For example:
- vars:
language: [French, German, Spanish]
input: file://path/to/inputs/*.txt
Using nunjucks templates
Use Nunjucks templates to exert additional control over your prompt templates, including loops, conditionals, and more.
Manipulating objects
In the above examples, vars
values are strings. But vars
can be any JSON or YAML entity, including nested objects. You can manipulate these objects in the prompt, which are nunjucks templates:
promptfooconfig.yaml:
tests:
- vars:
user_profile:
name: John Doe
interests:
- reading
- gaming
- hiking
recent_activity:
type: reading
details:
title: 'The Great Gatsby'
author: 'F. Scott Fitzgerald'
prompt.txt:
User Profile:
- Name: {{ user_profile.name }}
- Interests: {{ user_profile.interests | join(', ') }}
- Recent Activity: {{ recent_activity.type }} on "{{ recent_activity.details.title }}" by {{ recent_activity.details.author }}
Based on the above user profile, generate a personalized reading recommendation list that includes books similar to "{{ recent_activity.details.title }}" and aligns with the user's interests.
Here's another example. Consider this test case, which lists a handful of user and assistant messages in an OpenAI-compatible format:
tests:
- vars:
previous_messages:
- role: user
content: hello world
- role: assistant
content: how are you?
- role: user
content: great, thanks
The corresponding prompt.txt
file simply passes through the previous_messages
object using the dump filter to convert the object to a JSON string:
{{ previous_messages | dump }}
Running promptfoo eval -p prompt.txt -c path_to.yaml
will call the Chat Completion API with the following prompt:
[
{
"role": "user",
"content": "hello world"
},
{
"role": "assistant",
"content": "how are you?"
},
{
"role": "user",
"content": "great, thanks"
}
]
Escaping JSON strings
If the prompt is valid JSON, nunjucks variables are automatically escaped when they are included in strings:
tests:
- vars:
system_message: >
This multiline "system message" with quotes...
Is automatically escaped in JSON prompts!
{
"role": "system",
"content": "{{ system_message }}"
}
You can also manually escape the string using the nunjucks dump filter. This is necessary if your prompt is not valid JSON, for example if you are using nunjucks syntax:
{
"role": {% if 'admin' in message %} "system" {% else %} "user" {% endif %},
"content": {{ message | dump }}
}
Variable composition
Variables can reference other variables:
prompts:
- 'Write a {{item}}'
tests:
- vars:
item: 'tweet about {{topic}}'
topic: 'bananas'
- vars:
item: 'instagram about {{topic}}'
topic: 'theoretical quantum physics in alternate dimensions'
Tools and Functions
promptfoo supports tool use and function calling with OpenAI and Anthropic models, as well as other provider-specific configurations like temperature and number of tokens. For more information on defining functions and tools, see the OpenAI provider docs and the Anthropic provider docs.
Transforming outputs
The TestCase.options.transform
field is a Javascript snippet that modifies the LLM output before it is run through the test assertions.
It is a function that takes a string output and a context object:
transformFn: (output: string, context: {
prompt: {
// ID of the prompt, if assigned
id?: string;
// Raw prompt as provided in the test case, without {{variable}} substitution.
raw?: string;
// Prompt as sent to the LLM API and assertions.
display?: string;
};
vars?: Record<string, any>;
}) => void;
This is useful if you need to somehow transform or clean LLM output before running an eval.
For example:
# ...
tests:
- vars:
language: French
body: Hello world
options:
transform: output.toUpperCase()
# ...
Or multiline:
# ...
tests:
- vars:
language: French
body: Hello world
options:
transform: |
output = output.replace(context.vars.language, 'foo');
const words = output.split(' ').filter(x => !!x);
return JSON.stringify(words);
# ...
It also works in assertions, which is useful for picking values out of JSON:
tests:
- vars:
# ...
assert:
- type: equals
value: 'foo'
transform: output.category # Select the 'category' key from output json
Use defaultTest
apply a transform option to every test case in your test suite.
Transforms from separate files
Transform functions can be executed from external Python or Javascript files. For example:
defaultTest:
options:
transform: file://transform.js
Here's transform.js
:
module.exports = (output, context) => {
// context.vars, context.prompt
// ...
return output.toUpperCase();
};
Or the equivalent transform.py
:
def get_transform(output, context):
# context['vars'], context['prompt']
# ...
return output.upper()
Config structure and organization
For detailed information on the config structure, see Configuration Reference.
If you have multiple sets of tests, it helps to split them into multiple config files. Use the --config
or -c
parameter to run each individual config:
promptfoo eval -c usecase1.yaml
and
promptfoo eval -c usecase2.yaml
You can run multiple configs at the same time, which will combine them into a single eval. For example:
promptfoo eval -c my_configs/*
or
promptfoo eval -c config1.yaml -c config2.yaml -c config3.yaml
Loading tests from CSV
YAML is nice, but some organizations maintain their LLM tests in spreadsheets for ease of collaboration. promptfoo supports a special CSV file format.
prompts: [prompt1.txt, prompt2.txt]
providers: [openai:gpt-4o-mini, vertex:gemini-pro]
tests: tests.csv
promptfoo also has built-in ability to pull test cases from a Google Sheet. The easiest way to get started is to set the sheet visible to "anyone with the link". For example:
prompts: [prompt1.txt, prompt2.txt]
providers: [openai:gpt-4o-mini, vertex:gemini-pro]
tests: https://docs.google.com/spreadsheets/d/1eqFnv1vzkPvS7zG-mYsqNDwOzvSaiIAsKB3zKg9H18c/edit?usp=sharing
Here's a full example.
See Google Sheets integration for details on how to set up promptfoo to access a private spreadsheet.